这篇教程PyTorch一小时掌握之基本操作篇写得很实用,希望能帮到您。
创建数据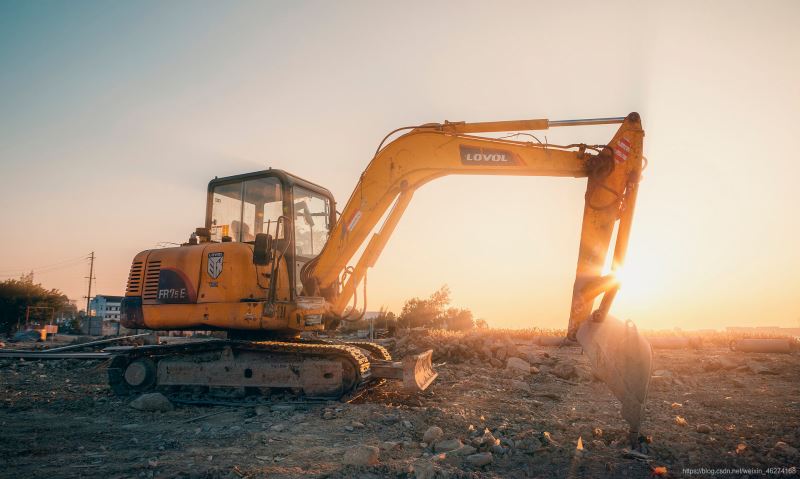
torch.empty()创建一个空张量矩阵. 格式: torch.empty(*size, *, out=None, dtype=None, layout=torch.strided, device=None, requires_grad=False, pin_memory=False, memory_format=torch.contiguous_format) → Tensor 参数: - size: 生成矩阵的形状, 必选
- dtype: 数据类型, 默认为 None
例子: # 创建一个形状为[2, 2]的矩阵a = torch.empty(2, 2)print(a)# 创建一个形状为[3, 3]的矩阵b = torch.empty(3, 3)print(b) 输出结果: tensor([[0., 0.], [0., 0.]]) tensor([[0., 0., 0.], [0., 0., 0.], [0., 0., 0.]])
torch.zeros()创建一个全零矩阵. 格式: torch.zeros(*size, *, out=None, dtype=None, layout=torch.strided, device=None, requires_grad=False) → Tensor 参数: - size: 生成矩阵的形状, 必选
- dtype: 数据类型, 默认为 None
例子: # 创建一个形状为[2, 2]的全零数组a = torch.zeros([2, 2], dtype=torch.float32)print(a)# 创建一个形状为[3, 3]的全零数组b = torch.zeros([3, 3], dtype=torch.float32)print(b) 输出结果: tensor([[0., 0.], [0., 0.]]) tensor([[0., 0., 0.], [0., 0., 0.], [0., 0., 0.]])
torch.ones()创建一个全一矩阵. 格式: torch.ones(*size, *, out=None, dtype=None, layout=torch.strided, device=None, requires_grad=False) → Tensor 参数: - size: 生成矩阵的形状, 必选
- dtype: 数据类型, 默认为 None
例子: # 创建一个形状为[2, 2]的全一数组a = torch.ones([2, 2], dtype=torch.float32)print(a)# 创建一个形状为[3, 3]的全一数组b = torch.ones([3, 3], dtype=torch.float32)print(b) 输出结果: tensor([[1., 1.], [1., 1.]]) tensor([[1., 1., 1.], [1., 1., 1.], [1., 1., 1.]])
torch.tensor()通过数据创建张量. 格式: torch.tensor(data, *, dtype=None, device=None, requires_grad=False, pin_memory=False) → Tensor 参数: - data: 数据 (数组, 元组, ndarray, scalar)
- dtype: 数据类型, 默认为 None
例子: # 通过数据创建张量array = np.arange(1, 10).reshape(3, 3)print(array)print(type(array))tensor = torch.tensor(array)print(tensor)print(type(tensor)) 输出结果: [[1 2 3] [4 5 6] [7 8 9]] <class 'numpy.ndarray'> tensor([[1, 2, 3], [4, 5, 6], [7, 8, 9]], dtype=torch.int32) <class 'torch.Tensor'>
torch.rand()创建一个 0~1 随机数的张量矩阵. 格式: torch.rand(*size, *, out=None, dtype=None, layout=torch.strided, device=None, requires_grad=False) → Tensor 参数: - size: 生成矩阵的形状, 必选
- dtype: 数据类型, 默认为 None
例子: # 创建形状为[2, 2]的随机数矩阵rand = torch.rand(2, 2)print(rand) 输出结果: tensor([[0.6209, 0.3424], [0.3506, 0.7986]])
数学运算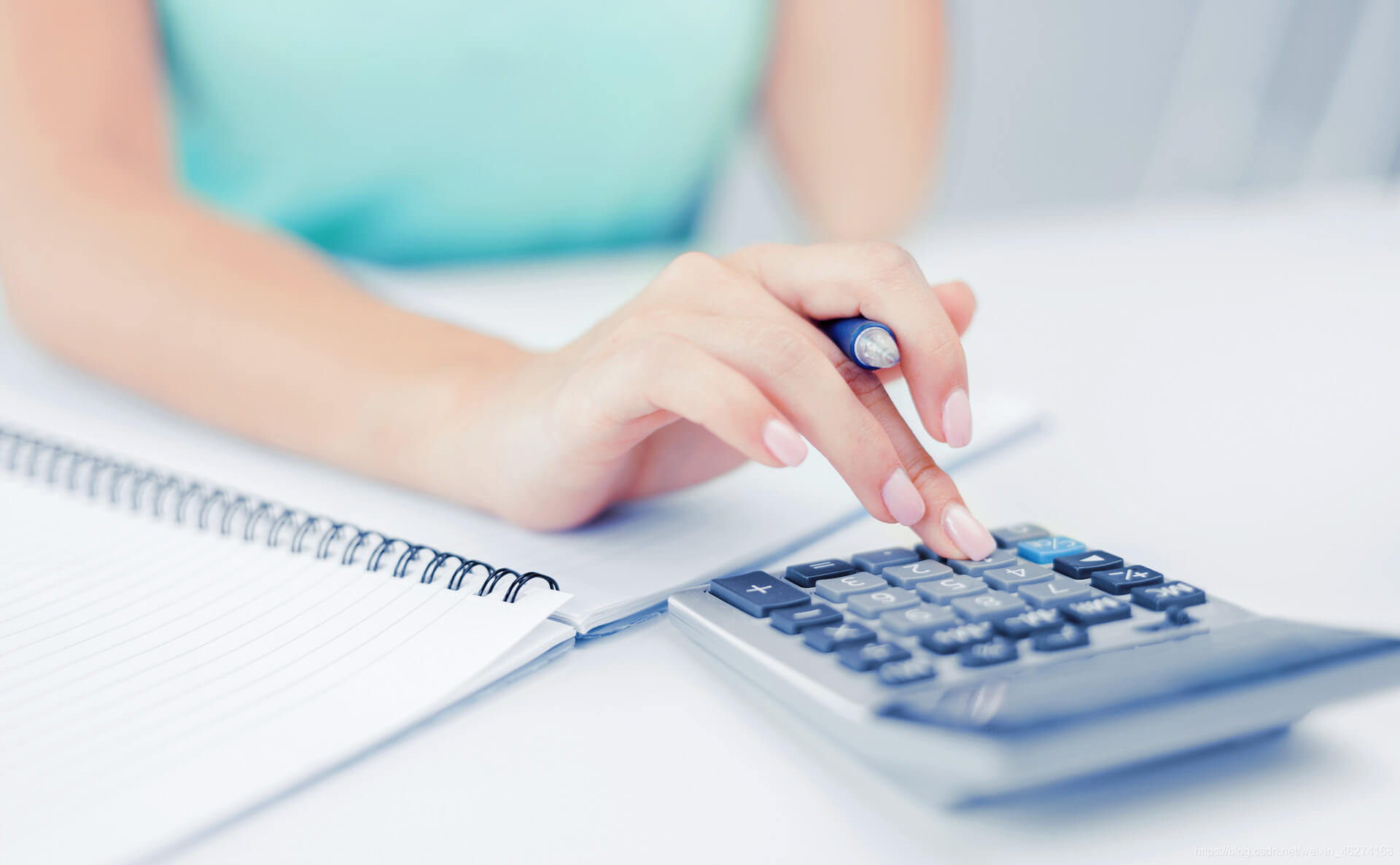
torch.add()返回相加的张量. 格式: torch.add(input, other, *, out=None) → Tensor 例子: # 张量相加input1 = torch.tensor([[1, 2], [3, 4]])print(input1)input2 = torch.tensor([[4, 3], [2, 1]])print(input2)output = torch.add(input1, input2)print(output) 输出结果: tensor([[1, 2], [3, 4]]) tensor([[4, 3], [2, 1]]) tensor([[5, 5], [5, 5]])
注: 相加的张量形状必须一致, 否则会报错.
torch.sub()返回相减的张量. 例子: # 张量相减input1 = torch.tensor([[1, 2], [3, 4]])print(input1)input2 = torch.tensor([[4, 3], [2, 1]])print(input2)output = torch.sub(input1, input2)print(output) 输出结果: tensor([[1, 2], [3, 4]]) tensor([[4, 3], [2, 1]]) tensor([[-3, -1], [ 1, 3]])
torch.matmul()例子: # 张量矩阵相乘input1 = torch.tensor([[1, 1, 1]])print(input1)input2 = torch.tensor([[3], [3], [3]])print(input2)output = torch.matmul(input1, input2)print(output) 输出结果: tensor([[1, 1, 1]]) tensor([[3], [3], [3]]) tensor([[9]])
索引操作索引 (index) 可以帮助我们快速的找到张量中的特定信息. 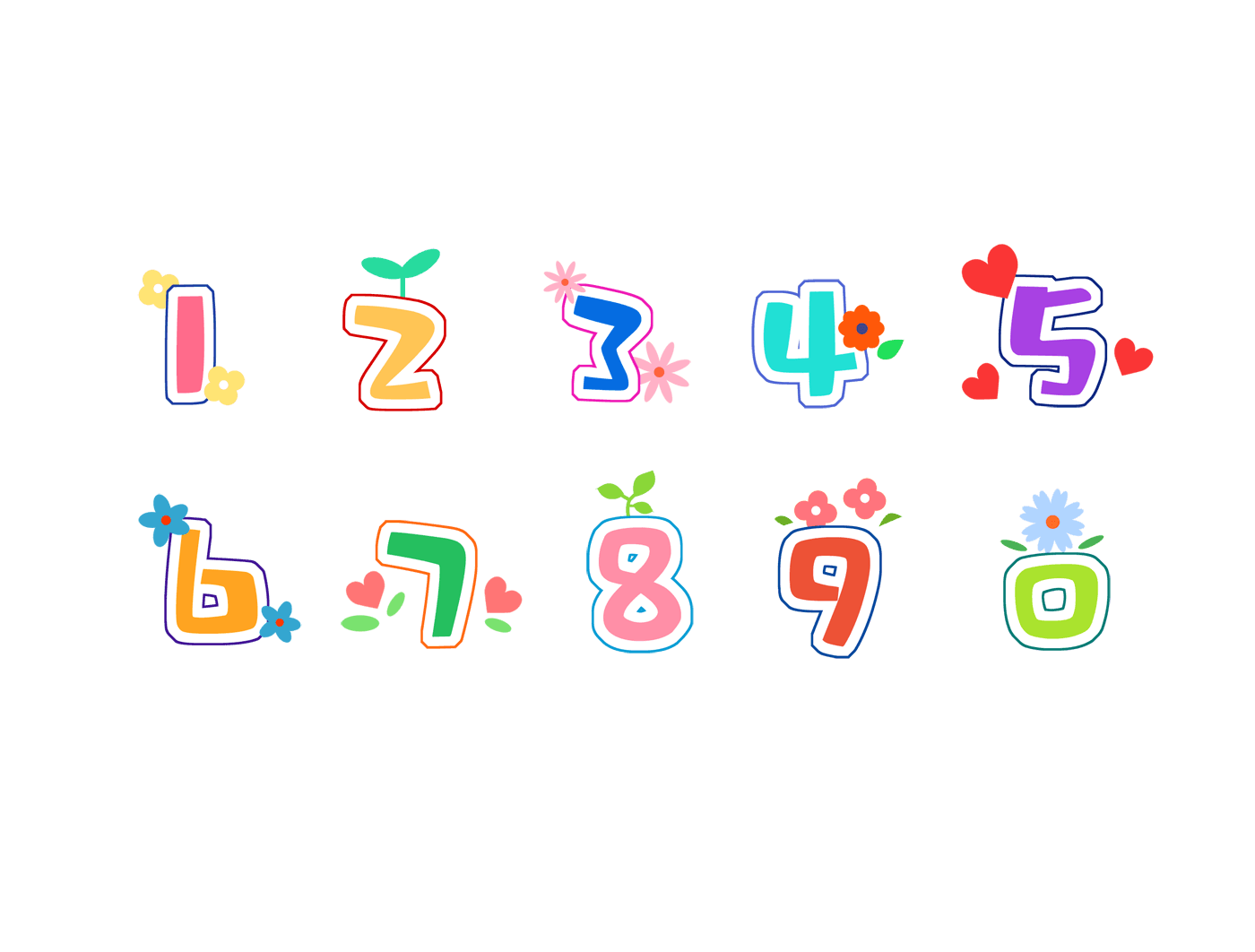
例子: # 简单的索引操作ones = torch.ones([3, 3])print(ones[: 2])print(ones[:, : 2]) 调试输出: tensor([[1., 1., 1.], [1., 1., 1.]]) tensor([[1., 1.], [1., 1.], [1., 1.]])
到此这篇关于PyTorch一小时掌握之基本操作篇的文章就介绍到这了,更多相关PyTorch基本操作内容请搜索51zixue.net以前的文章或继续浏览下面的相关文章希望大家以后多多支持51zixue.net! PyTorch一小时掌握之图像识别实战篇 教你如何在Pygame 中移动你的游戏角色 |