这篇教程python脚本之如何按照清晰度对图片进行分类写得很实用,希望能帮到您。
原理在《Analysis of focus measure operators for shape-from-focus》[2013 Pertuz et al.]。 在这篇文献中,Pertuz 等人论述了近 36 种不同的图片清晰度评价(focus measure)方法。 其中一种方式比较简单,就是使用拉普拉斯方差算法,直接得到一个浮点数方差表征清晰度。 事先设定一个阈值,如果某图片的清晰度低于预先定义的阈值,那么该图片就可以被认为是模糊的。高于阈值,就不是模糊的,或者说是清晰的。 此算法基于以下假设:如果图片具有较高方差,那么它就有较广的频响范围,代表着正常,聚焦准确的图片。 总结:正常图像中边界清晰,经拉普拉斯计算后方差较大;模糊图像边界信息少,方差小
代码import osimport cv2import shutil# 计算图片清晰度def getImageVar(img): res=0 for i in cv2.split(img): # 对图片用 3x3 拉普拉斯算子做卷积得到边缘 计算出方差,并最后返回。 # 函数求完导数后会有负值,还有会大于255的值。而原图像是uint8,即8位无符号数,所以建立的图像位数不够,会有截断。因此要使用64位有符号的数据类型,即 cv2.CV_64F。 # 再用var函数求方差 res+=cv2.Laplacian(i, cv2.CV_64F).var() return res/3# 读取某个文件夹下面的所有文件名 返回listdef get_all_file(path): all_file=[] for i in os.listdir(path): file_name=os.path.join(path,i) all_file.append(file_name) return all_fileinput_path=input("请输出目标文件夹:")threshold=int(input("请输入清晰度阈值:"))orign_path=os.path.join(os.path.abspath('.'),'img')resoure_file_path = os.path.join(orign_path, input_path) # 图片所在文件夹vage_file_path=os.path.join(orign_path,'vague')clear_file_path=os.path.join(orign_path,'clear')if not os.path.exists(vage_file_path): os.mkdir(vage_file_path)if not os.path.exists(clear_file_path): os.mkdir(clear_file_path)all_img_path=get_all_file(resoure_file_path)for img_path in all_img_path: img_name=img_path.split('//')[-1] dst=os.path.join(vage_file_path,img_name) if getImageVar(cv2.imread(img_path))>threshold: dst = os.path.join(clear_file_path, img_name) shutil.copyfile(img_path, dst) 打包成exe:
使用首先准备一个img 文件夹和img_cls.exe 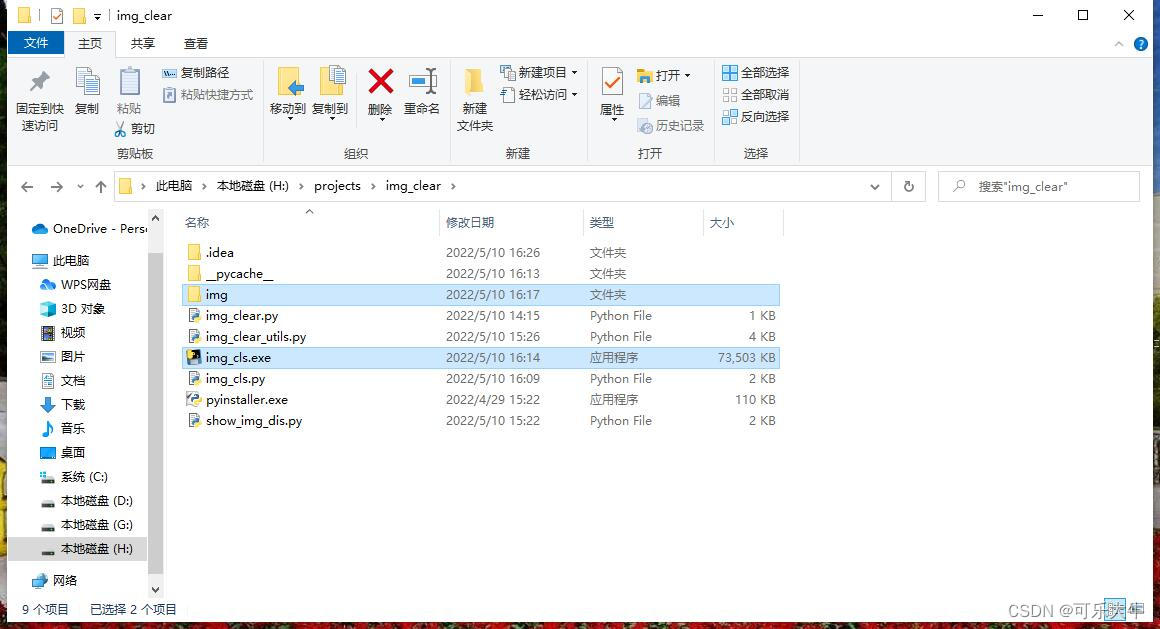
img 文件夹中包含存放需要分类的图片的文件夹自定义(如test_img)以及将来的两个结果文件夹vague 和clear
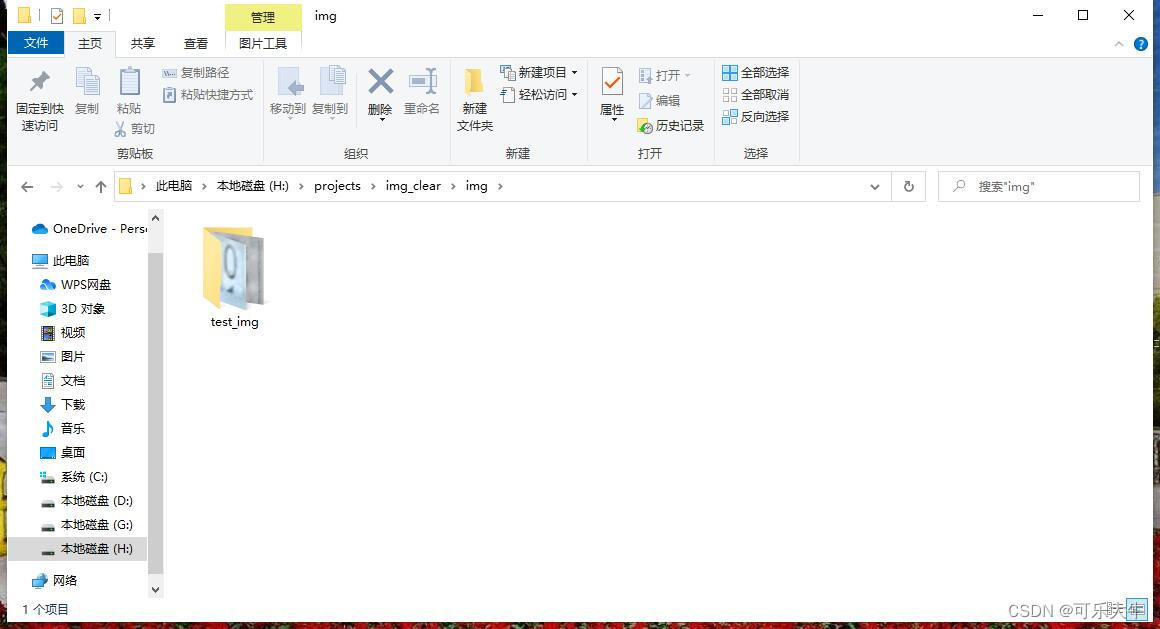
双击执行exe文件,输入自定义和清晰度门限(高于阈值是清晰,低于阈值是模糊) 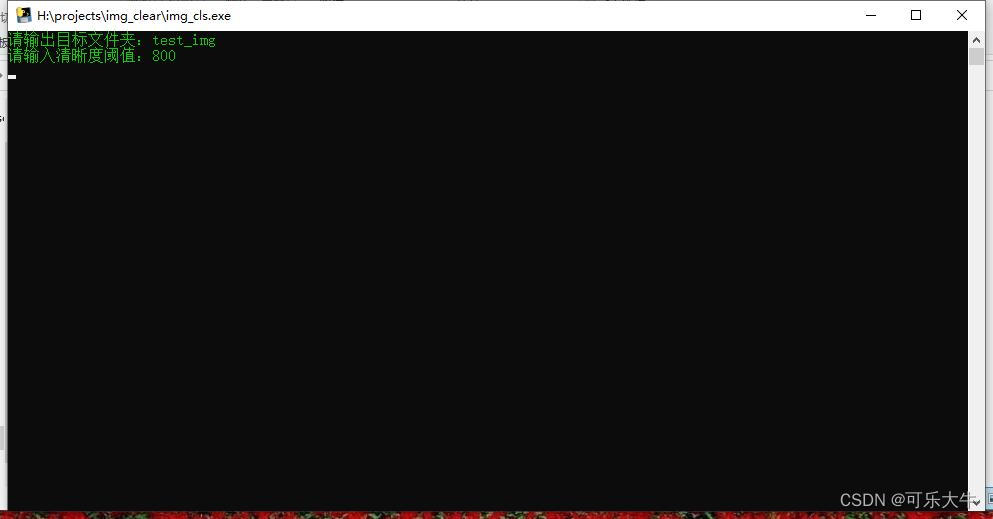
结束之后得到: 两个分类文件夹(复制过来的 原图片没动) 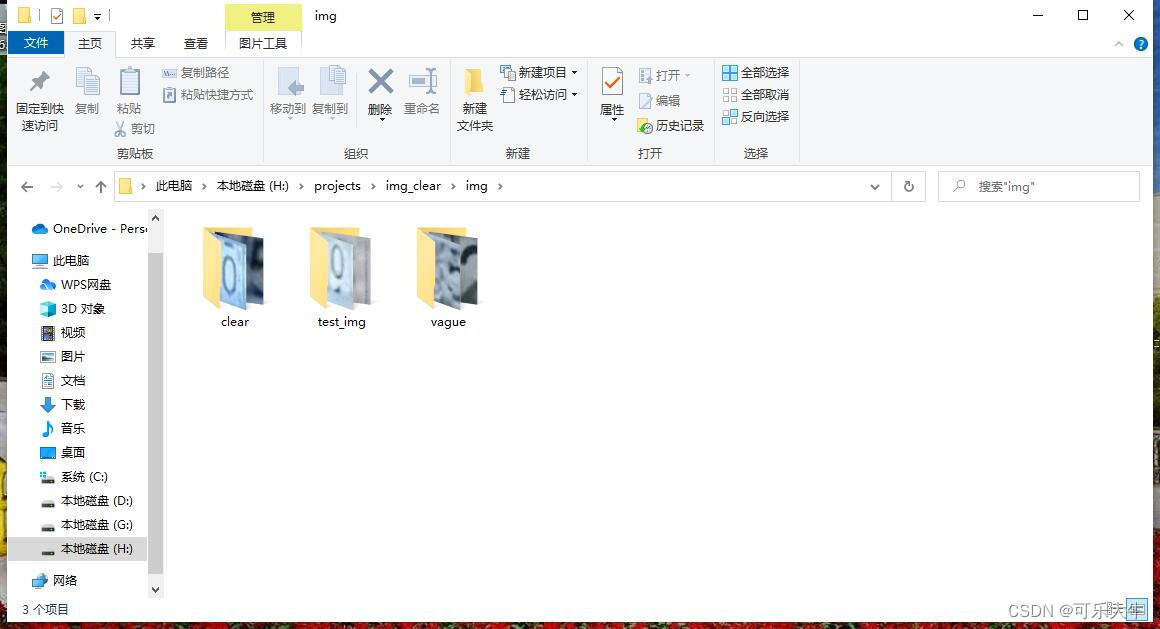
总结以上为个人经验,希望能给大家一个参考,也希望大家多多支持wanshiok.com。 Python安装第三方库的方法(pip/conda、easy_install、setup.py) Python虚拟机中描述器的王炸应用分享 |